If there’s one thing that the COVID-19 pandemic has brought into sharp relief that the vast majority of physicians and scientists either didn’t appreciate or downplayed as unimportant is just how easily people can be led into believing in conspiracy theories and science denial such as antivaccine pseudoscience, including highly educated professionals, such as physicians and scientists. In the slightly less than two and a half years since COVID-19 was declared a pandemic, just at this blog alone we’ve written about many examples whom, before the pandemic, we had never heard of, such as Peter McCullough, Michael Yeadon, Robert Malone, Simone Gold, and Geert Vanden Bossche. Others were academics with whom I had not been familiar, such as the Great Barrington Declaration (GBD) authors Martin Kulldorff, Jay Bhattacharya, and Sunetra Gupta, all of whom had been well-respected before their pandemic disinformation heel turn first to COVID-19 contrarianism and then to outright antivaccine disinformation. There were also scientists with whom we were familiar, making their turn to contrarian but whose turn to contrarianism and science denial actually shocked me, such as John Ioannidis, and ones whose heel turn with respect to science surprised me less, such as Vinay Prasad. Throughout the pandemic, I was never surprised when scientists and physicians who had been antivaccine prior to the pandemic (e.g., James Lyons-Weiler, Paul Thomas, Sherri Tenpenny, Andrew Wakefield, and Joe Mercola) immediately pivoted to COVID-19 minimization and antivaccine disinformation weaponized against COVID-19 vaccines, but some of the others genuinely puzzled me with how easily they had “turned” into spreaders of misinformation.
Looking over the list of these people and others, one can find a number of commonalities shared by some, but not all, of the contrarians listed above. . First, most of them have an affinity towards at least libertarian-leaning politics. Second, few of them have any significant expertise in infectious disease or infectious disease epidemiology. One exception is Sunetra Gupta, co-author of the GBD, is a British infectious disease epidemiologist and a professor of theoretical epidemiology at the Department of Zoology, University of Oxford. She’s a modeller, but early in the pandemic her group’s modeling, which concluded that by March 2020 more than half of the UK population might have been infected with the novel coronavirus and therefore “natural herd immunity” was within reach, disagreed markedly with the scientific consensus, which might have played a role. (Instead of wondering where she might have gone wrong, Gupta appears to have doubled down.) However, most of the others above have little or no directly relevant experience in infectious disease, infectious disease epidemiology, virology, immunology, vaccine development, or molecular biology. Yet during the pandemic they became famous—or at least Internet influencers, although a number of them—I’m looking at you, Kulldorff and Bhattacharya—have shown up fairly regularly on Fox News to attack public health interventions and mandates targeting the pandemic.
So what is the common denominator? A recent study published in ScienceAdvances last month suggests at least one reason. Steve Novella wrote about it on his personal blog the week that it was published, but I wanted to add my take and integrate it into a more general discussion of how people—including some who, one would think, should know better based on their education and profession—can so easily fall prey to science denial and conspiracy theories. As Steve noted in his post, for most people other than experts in relevant fields, a very good “first approximation of what is most likely to be true is to understand and follow the consensus of expert scientific opinion.” As he put it, that’s just probability. It doesn’t mean that the experts are always right or that there’s no role for minority—or even fringe±—opinions. Rather, as Steve put it:
It mostly means that non-experts need to have an appropriate level of humility, and at least a basic understanding of the depth of knowledge that exists. I always invite people to consider the topic they know the best, and consider the level of knowledge of the average non-expert. Well, you are that non-expert on every other topic.
It is that humility that is lacking in these people (at least), as suggested by the title of the study, Knowledge overconfidence is associated with anti-consensus views on controversial scientific issues.
Overconfidence versus scientific consensus
The SBM crew has consistently emphasized the need for humility in approaching science. I would add that this need is not limited to science in which one is an expert. For instance, ostensibly I’m an expert in breast cancer, which means that I know an awful lot about the biology of breast cancer and its clinical behavior. However, I am a surgical oncologist, not a medical oncologist; I would not presume to tell my medical oncology colleagues which chemotherapy or targeted therapy drugs they should give my patients after I operate on them, and, I would hope, they don’t presume to tell me who is a surgical candidate, what operation to perform, or how to perform it. Similarly, based on education, experience, and publications, I can be considered an expert in some areas of basic and translational science, such as breast cancer biology related to a certain class of glutamate receptors, tumor angiogenesis, and other areas in which I’ve done research and published.
Finally, after nearly 20 years of studying anti-science misinformation, the antivaccine movement, and conspiracy theories in general, I like to think that I have a certain level of expertise in these areas. That’s why, when COVID-19 first hit, I was careful about making declarations and tended to stick with the scientific consensus unless new evidence suggested that I should do otherwise. However, I did immediately recognize the same sorts of science denial, antivaccine tropes, and conspiracy theories that I had long written about before the pandemic being applied to the pandemic, which is what I wrote about, such as early claims that SARS-CoV-2 is a bioweapon and misuse of the Vaccine Adverse Events Recording System (VAERS) database. The last of these many of us had been predicting before COVID-19 vaccines received emergency use authorizations (EUAs) from the FDA and I wrote about within weeks of the rollout of the vaccines, as antivaxxers were already doing what they had long done for vaccines and autism, miscarriages, and sudden infant death syndrome (SIDS). I recount these incidents mainly to demonstrate how, if you don’t know the sorts of conspiracy theories that have long spread among antivaxxers, you wouldn’t know that everything old is new again, there is nothing new under the sun, and antivaxxers tend just to recycle and repurpose old conspiracy theories to new vaccines, like COVID-19 vaccines. If many of the people above, most of whom self-righteously proclaim themselves “provaccine,” did know these tropes beforehand, I like to think that some of them might not have turned antivax.
Let’s move on to the study, which comes from investigators from Portland State University, the University of Colorado, Brown University, and the University of Kansas. From the title of the study, you might think that this is primarily about the Dunning-Kruger effect, a cognitive bias well known among skeptics in which people wrongly overestimate their knowledge or ability in a specific area. While there are criticisms of the Dunning-Kruger model, it has generally held up pretty well as one potential explanation of how people come to believe anti-consensus views.
This particular study, the authors point one important point out before they describe their methods and results:
Opposition to the scientific consensus has often been attributed to nonexperts’ lack of knowledge, an idea referred to as the “deficit model” (7, 8). According to this view, people lack specific scientific knowledge, allowing attitudes from lay theories, rumors, or uninformed peers to predominate. If only people knew the facts, the deficit model posits, then they would be able to arrive at beliefs more consistent with the science. Proponents of the deficit model attempt to change attitudes through educational interventions and cite survey evidence that typically finds a moderate relation between science literacy and pro-consensus views (9–11). However, education-based interventions to bring the public in line with the scientific consensus have shown little efficacy, casting doubt on the value of the deficit model (12–14). This has led to a broadening of psychological theories that emphasize factors beyond individual knowledge. One such theory, “cultural cognition,” posits that people’s beliefs are shaped more by their cultural values or affiliations, which lead them to selectively take in and interpret information in a way that conforms to their worldviews (15–17). Evidence in support of the cultural cognition model is compelling, but other findings suggest that knowledge is still relevant. Higher levels of education, science literacy, and numeracy have been found to be associated with more polarization between groups on controversial and scientific topics (18–21). Some have suggested that better reasoning ability makes it easier for individuals to deduce their way to the conclusions they already value [(19) but see (22)]. Others have found that scientific knowledge and ideology contribute separately to attitudes (23, 24).
Recently, evidence has emerged, suggesting a potentially important revision to models of the relationship between knowledge and anti-science attitudes: Those with the most extreme anti-consensus views may be the least likely to apprehend the gaps in their knowledge.
This is, as Steve described it, a “super Dunning-Kruger.” The authors, however, describe existing research and what they think that their research contributes to general knowledge thusly:
These findings suggest that knowledge may be related to pro-science attitudes but that subjective knowledge—individuals’ assessments of their own knowledge—may track anti-science attitudes. This is a concern if high subjective knowledge is an impediment to individuals’ openness to new information (30). Mismatches between what individuals actually know (“objective knowledge”) and subjective knowledge are not uncommon (31). People tend to be bad at evaluating how much they know, thinking they understand even simple objects much better than they actually do (32). This is why self-reported understanding decreases after people try to generate mechanistic explanations, and why novices are poorer judges of their talents than experts (33, 34). Here, we explore such knowledge miscalibration as it relates to degree of disagreement with scientific consensus, finding that increasing opposition to the consensus is associated with higher levels of knowledge confidence for several scientific issues but lower levels of actual knowledge. These relationships are correlational, and they should not be interpreted as support for any one theory or model of anti-scientific attitudes. Attitudes like these are most likely driven by a complex interaction of factors, including objective and self-perceived knowledge, as well as community influences. We speculate on some of these mechanisms in the general discussion.
The authors do this through five studies estimating opposition to scientific consensus, as well as objective knowledge and subjective knowledge of these topics:
In studies 1 to 3, we examine seven controversial issues on which there is a substantial scientific consensus: climate change, GM foods, vaccination, nuclear power, homeopathic medicine, evolution, and the Big Bang theory. In studies 4 and 5, we examine attitudes concerning COVID-19. Second, we provide evidence that subjective knowledge of science is meaningfully associated with behavior. When the uninformed claim they understand an issue, it is not just cheap talk, and they are not imagining a set of “alternative facts.” We show that they are willing to bet on their ability to perform well on a test of their knowledge (study 3).
The key part of the study is portrayed in this graph, Figure 1:
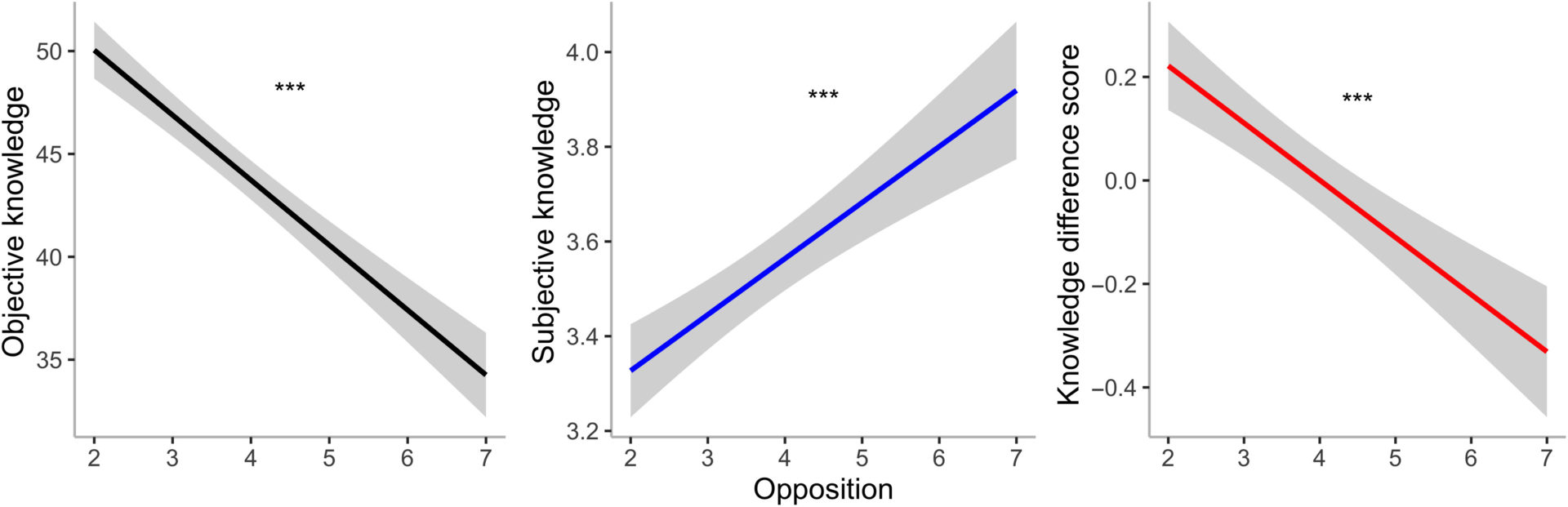
Fig. 1. Overall across-issue model predictions of relationships between opposition and objective knowledge, subjective knowledge, and the knowledge difference score, with 95% confidence interval bands.
Higher levels of opposition to a scientific consensus are associated with lower levels of actual scientific knowledge, higher self-assessments of knowledge, and more knowledge overconfidence (operationalized here as the increasing negative magnitude of each respondent’s knowledge difference score). ***P < .001.[/caption]
Because there could be differences based on topic, the authors then broke their results down individual contentious area:
[caption id="attachment_81132" align="aligncenter" width="1920"] Fig. 2. The relationship between opposition and subjective and objective knowledge for each of the seven scientific issues, with 95% confidence bands.
In general, opposition is positively associated with subjective knowledge and negatively associated with objective knowledge, but not for all issues.
The authors note that the relationship between opposition to the scientific consensus and objective knowledge was negative and significant for all episodes other than climate change, while the relationship between opposition and subjective knowledge was positive for all issues other than climate change, Big Bang, and evolution, where the relationship failed to achieve statistical significance.
The authors also noted one interesting effect, specifically that of political polarization, reporting that, for more politically polarized issues, the relation between opposition and objective knowledge is less negative than for less polarized issues and that the relation between opposition and subjective knowledge is less positive. One way that this sort of result might be interpreted is that advocates who take on an anti-consensus view go out of their way to learn background information about the subject that they oppose. However, in this case knowledge doesn’t lead to understanding, but rather to more skilled at motivated reasoning; i.e., picking and choosing information and data that support one’s preexisting beliefs.
Because another issue is that study subjects with different levels of opposition to a scientific consensus might interpret their subjective knowledge differently, noting that “opponents may claim that they understand an issue but acknowledge that their understanding does not reflect the same facts as the scientific community” and that this “could explain the disconnect between their subjective knowledge rating and their ability to answer questions based on accepted scientific facts.” So to test this, the authors developed a measure of knowledge confidence designed to remove this ambiguity by designing a measure of knowledge confidence that incentivized participants to report their genuine beliefs. Specifically, subjects were given an opportunity to earn a bonus payment by betting on their ability to score above average on the objective knowledge questions or to take a smaller guaranteed payout, with betting indicating greater knowledge confidence.
As the authors predicted the results were:
As opposition to the consensus increased, participants were more likely to bet but less likely to score above average on the objective knowledge questions, confirming our predictions. As a consequence, more extreme opponents earned less. Regression analysis revealed that there was a $0.03 reduction in overall pay with each one-unit increase in opposition [t(1169) = −8.47, P< 0.001]. We also replicated the effect that more opposition to the consensus is associated with higher subjective knowledge [βopposition = 1.81, t(1171) = 7.18, P < 0.001] and lower objective knowledge [both overall science literacy and the subscales; overall science literacy model βopposition = −1.36, t(1111.6) = −16.28, P < 0.001; subscales model βopposition = −0.19, t(1171) = −10.38, P < 0.001]. Last, participants who chose to bet were significantly more opposed than nonbetters [βbet = 0.24, t(1168.7) = 2.09, P = 0.04], and betting was significantly correlated with subjective knowledge [correlation coefficient (r) = 0.28, P < 0.001], as we would expect if they are related measures. All effects were also significant when excluding people fully in line with the consensus (see the Supplementary Materials for analysis).
Finally, the authors applied similar methods to questions of whether or not study subjects would take the COVID-19 vaccine (study #4) and attitudes towards COVID-19 public health interventions (study #5). Study #4 replicated the results of previous studies. There was an interesting curveball in study #5, though, questions on how much study participants think that scientists know about COVID-19, and the results were telling:
To validate the main finding, we split the sample into those who rated their own knowledge higher than scientists’ knowledge (28% of the sample) and those who did not. This dichotomous variable was also highly predictive of responses: Those who rated their own knowledge higher than scientists’ were more opposed to virus mitigation policies [M = 3.66 versus M = 2.66, t(692) = −12, P < 0.001, d = 1.01] and more noncompliant with recommended COVID-mitigating behaviors [M = 3.05 versus M = 2.39, t(692) = −9.08, P < 0.001, d = 0.72] while scoring lower on the objective knowledge measure [M = 0.57 versus M = 0.67, t(692) = 7.74, P < 0.001, d = 0.65]. For robustness, we replicated these patterns in identical models controlling for political identity and in models using a subset scale of the objective knowledge questions that conservatives were not more likely to answer incorrectly. All effects remained significant. Together, these results speak against the possibility that the relation between policy attitudes and objective knowledge on COVID is completely explained by political ideology (see the Supplementary Materials for all political analyses).
This could also suggest why certain individuals who self-identified as liberal or progressive have fallen for COVID-19 contrarianism:
Results from five studies show that the people who disagree most with the scientific consensus know less about the relevant issues, but they think they know more. These results suggest that this phenomenon is fairly general, although the relationships were weaker for some more polarized issues, particularly climate change. It is important to note that we document larger mismatches between subjective and objective knowledge among participants who are more opposed to the scientific consensus. Thus, although broadly consistent with the Dunning-Kruger effect and other research on knowledge miscalibration, our findings represent a pattern of relationships that goes beyond overconfidence among the least knowledgeable. However, the data are correlational, and the normal caveats apply.
Before I move on to the general public, personally, I can’t help but wonder if these results have particular relevance in suggesting how scientists and physicians who should know better could come to hold anti-consensus views so strongly. For example, let’s look at the cases of John Ioannidis and Vinay Prasad. John Ioannidis made his name as a “meta-scientist” or critic of science. His publication record covers documentation of deficiencies in the scientific evidence for a broad number of scientific subject areas, ranging from the effect of nutrition on cancer to, well, pretty much all clinical science. Not long after the pandemic hit I started wondering what the heck happened to him and he started abusing science to attack scientists holding consensus views about COVID-19 as “science Kardashians,” I had already started becoming uncomfortable with his attitude as being more knowledgeable about everything, as evidenced by his arguing that the NIH rewards “conformity” and “mediocrity” when awarding research grants. I would speculate—perhaps even argue—that the overconfidence in his own knowledge described in this study had already infected Ioannidis before the pandemic.
Similarly, before the pandemic Prasad had made his name criticizing the evidence base for oncology interventions. (He is an academic medical oncologist.) In particular, he was known for documenting what he referred to as “medical reversals,” when an existing medical practice is shown to be no better than a “lesser” therapy. Both Steve and I discussed this concept at the time and both agreed that, while Prasad’s work produced some useful observations, it also missed a fair amount of nuance. By just before the pandemic, I note that Prasad had taken to criticizing those of us who were combatting pseudoscience in medicine as, in essence, wasting our medical skills, denigrating such activities as being below him, as a pro basketball star “dunking on a 7′ hoop.” As with Ioannidis, I would speculate—perhaps even argue—that the overconfidence in his own knowledge described in this study had already infected Prasad before the pandemic. One could easily speculate that all of the “COVID contrarian doctors” who went anti-consensus—some of whom even became antivaccine— likely shared this overconfidence before the pandemic.
One thing this study doesn’t address, though, that I’ve been wondering about, and that’s the role of social affirmation in reinforcing anti-consensus views and even radicalizing such “contrarian doctors” further into anti-science views. Vinay Prasad and many of the other physicians and scientists I listed have large social media presences and legions of adoring fans. Ioannidis, although he frequently humble brags about not being on social media, does receive lots of invitations to be interviewed in more conventional media from all over the world, and the reasons are his pre-pandemic fame as the most published living scientists and his COVID-19 contrarian views. Then, for many of these doctors, there are financial rewards. A number of them have Substacks in which they monetize their contrarian and science-denying views.
These would be good topics for other studies. In the meantime, let’s move on to the general public that consumes—and is influenced by—their misinformation.
Who is persuadable?
The Science Advances study notes something that skeptics have known for a long time. It isn’t (just) lack of information that drives science denial. It’s more than that, which is why, in and of itself, trying to drive out bad information with good information generally doesn’t work very well:
The findings from these five studies have several important implications for science communicators and policymakers. Given that the most extreme opponents of the scientific consensus tend to be those who are most overconfident in their knowledge, fact-based educational interventions are less likely to be effective for this audience. For instance, The Ad Council conducted one of the largest public education campaigns in history in an effort to convince people to get the COVID-19 vaccine (43). If individuals who hold strong antivaccine beliefs already think that they know all there is to know about vaccination and COVID-19, then the campaign is unlikely to persuade them.
Instead of interventions focused on objective knowledge alone, these findings suggest that focusing on changing individuals’ perceptions of their own knowledge may be a helpful first step. The challenge then becomes finding appropriate ways to convince anti-consensus individuals that they are not as knowledgeable as they think they are.
This is not an easy task. I frequently point out that hard core antivaxxers, for instance, are generally not persuadable. Indeed, I liken their antivaccine views—or other anti-consensus views—to religion or political affiliation, beliefs intrinsic to their identities and every bit as difficult to change as religion or political orientation. In other words, it’s possible to change such views, but the effort required and failure rate are both too high to make these people a target of science communication. As a corollary to this principle, I’ve long argued that it is the fence sitters who are the most likely to have their minds changed, or to be “inoculated” (if you will) against misinformation.
Last week, a study from authors at the Santa Fe Institute was published in the same journal suggesting that I should be rather humble, as I might not be entirely on the right track, in that people do tend to shape their beliefs according to their social networks and existing moral belief systems. The authors note, as I frequently do:
Skepticism toward childhood vaccines and genetically modified food has grown despite scientific evidence of their safety. Beliefs about scientific issues are difficult to change because they are entrenched within many interrelated moral concerns and beliefs about what others think.
Again, the reason many people gravitate to anti-consensus views with respect to specific scientific conclusions (e.g., vaccine safety and effectiveness) involves social moral beliefs and concerns and personal ideology. To determine who is most susceptible to belief change, the authors, Information alone, although a necessary precondition to change minds, is usually not sufficient to change minds. I would also argue that it’s equally important to identify whose minds are changeable with achievable effort.
To boil this research down, the authors looked at two scientific issues, vaccines and genetically modified organisms (GMOs), using this rationale:
In this paper, we consider attitudes toward GM food and childhood vaccines as networks of connected beliefs (7–9). Inspired by statistical physics, we are able to precisely estimate the strength and direction of the belief network’s ties (i.e., connections), as well as the network’s overall interdependence and dissonance. We then use this cognitive network model to predict belief change. Using data from a longitudinal nationally representative study with an educational intervention, we test whether our measure of belief network dissonance can explain under which circumstances individuals are more likely to change their beliefs over time. We also explore how our cognitive model can shed light on the dynamic nature of dissonance reduction that leads to belief change. By combining a unifying predictive model with a longitudinal dataset, we expand upon the strengths of earlier investigations into science communication and belief change dynamics, as we describe in the next paragraphs.
In brief, investigators constructed a cognitive belief network model to predict how the beliefs of a group of almost 1,000 people who were at least somewhat skeptical about the efficacy of genetically modified foods and childhood vaccines would change as the result of an educational intervention. Using a nationally representative longitudinal study of beliefs about GM food and vaccines carried out at four different times over three waves of data collection (once in the first and third waves and twice in the second wave, before and after an intervention). During the second wave, the authors presented subjects with an educational intervention on the safety of GM food and vaccines, quoting reports from the National Academies of Sciences. Participants were divided into five experimental groups for the GM food study and four experimental groups for the study of childhood vaccines, with one control condition in each study in which participants did not receive any intervention. All experimental conditions received the same scientific message about safety with a different framework. The results were then analyzed using the cognitive network model developed, to look for how beliefs would change in response to the educational intervention.
To sum it up, those who had a lot of dissonance in their interwoven network of beliefs were more likely to change their beliefs after viewing the messaging, although not necessarily in accordance with the message. Overall, the authors found that people are driven to lower their cognitive dissonance through belief change. As the authors put it in an interview for their press release in which they commented on how people with little dissonance showed little change in their beliefs after the intervention and those with more dissonance were more likely to show more change:
“For example, if you believe that scientists are inherently trustworthy, but your family and friends tell you that vaccines are unsafe, this is going to create some dissonance in your mind,” van der Does says. “We found that if you were already kind of anti-GM foods or vaccines to begin with, you would just move more towards that direction when presented with new information even if that wasn’t the intention of the intervention.”
What this study suggests is that targeting such people with science communication can be a two-edged sword in that people with high dissonance will try to reduce their dissonance. Unfortunately, reducing that dissonance might not go in the direction that you think it will. They might reduce their dissonance by moving towards accepting scientific consensus with respect to scientific issues that are contentious among the public, such as vaccines and GM foods, or they might move further into conspiracy:
All in all, we found that network dissonance, belief change, and interdependence relate to each other over time, in line with our model assumptions. Interventions aimed at changing people’s beliefs led to a reconfiguration of beliefs that allowed people to move to lower network dissonance states and more consistent belief networks. However, these reconfigurations were not always in line with the objective of the intervention and sometimes even reflected a backlash.
And:
Individuals are motivated to reduce the dissonance between beliefs and reconfigure their beliefs to allow for lower dissonance. Such a reconfiguration can be, but is not necessarily, in line with the aim of the intervention. The direction in which individuals change their beliefs depends not only on the intervention but also on the easiest way for individuals to reduce their dissonance. This finding also goes beyond the classic finding that inducing dissonance leads to belief change (41–43) by showing that providing individuals with new information interacts with dissonances in their belief network. Individuals with low dissonance are unlikely to change at all, whereas individuals with high dissonance can change in both directions.
So the real question, if this research holds up, is: How do we identify people with a high degree of dissonance who won’t reduce their dissonance in response to a pro-science message by moving deeper into antiscience beliefs and conspiracy theories? More importantly, how do we craft messages that make it less likely that these people will adjust their anti-consensus beliefs in the direction that we want them to, rather than doubling down? Again, it is clear that those who are the most certain and have the least dissonance are the least likely to change their views in response to science communication.
Putting it all together
How does one put the results of these two studies together and combine them with what is already known? From my perspective, I see a couple of very important takeaway points. First, as Steve has said, humility is key. One is tempted to quote Richard Feynman, who famously said, “The first principle is that you must not fool yourself and you are the easiest person to fool.” My colleagues who “go crank” almost all have forgotten Feynman’s warning.
Indeed, those who follow my Twitter feed might have noticed me saying things like this lately:
Yep. The first step towards becoming a skeptic (and not a pseudoskeptic) is to admit to yourself—and really mean it—that you, too, can fall for these sorts of conspiracy theories and errors of thinkings. https://t.co/dgAs0GitTI
— David Gorski, MD, PhD (@gorskon) August 12, 2022
The moment you think you're immune to conspiracy theories, you're doomed. Unfortunately, a lot of self-identified movement skeptics—some quite prominent in the movement—seem not to have taken these messages to heart, especially lately about, for example, transgender medicine./end
— David Gorski, MD, PhD (@gorskon) August 12, 2022
I won’t name those skeptics here, but the warning above especially applies to people like John Ioannidis, who seems to think that, having spent over a quarter century documenting problems with biomedical research, he is somehow immune to the same errors in thinking that lead others astray. Then there’s Vinay Prasad, who not only doesn’t think that such errors in thinking are important enough to trouble his massive brain with, but has expressed outright contempt towards those of us who do and act upon that belief by combatting medical missinformation. It is not difficult to see how two men who built their academic careers on “meta-science” and, in essence, commenting on deficiencies in science, might come to think that they know better than the scientists with relevant topic-specific expertise, rather than more general expertise about scientific methodology.
Ideology also plays a role. Martin Kulldorff is an excellent example of this. He clearly almost certainly must have had preexisting libertarian views towards collective action led by government as anathema. I say this based on how easily Jeffrey Tucker of the “free market” think tank American Institute for Economic Research enticed him to come to its headquarters, where, having found his people, Kulldorff wanted to hold the conference at which the GBD was birthed more urgently than even Tucker did. Ever since then, Kulldorff, along with his fellow GBD author Jay Bhattacharya, has been slipping further and further from science and deeper and deeper into conspiracy theory and antivaccine advocacy.
As for the rest, most of them weren’t academic, and those who tended to be either adjunct clinical faculty or already flirting with crankdom. It isn’t hard to believe that what drew them into COVID-19 contrarianism was the ego boost from the attention that they got for promoting views that went against the mainstream. I’ll reiterate right here that there is nothing inherently wrong about challenging the mainstream. What matters is that you have the goods—the evidence and/or logic—to back up that challenge. That’s what differentiates legitimate scientists expressing nonmainstream views from the cranks.
Then, of course, the role of grift cannot be underemphasized. As I like to say, ideology is key, but it’s often also always about the grift. Just look at America’s Frontline Doctors if you don’t belief me.
As for reaching the public, what these studies suggest is that science communication is complex and far more difficult than most people appreciate. While it’s undeniably true that the more certain that, for example, an antivaxxer is, the less likely anyone is going to be able to change their mind, predicting who will be persuadable and won’t react to science communication by moving deeper into conspiracy and crafting messages to minimize that possibility are very difficult. The more I learn about this area, the less I feel confident that I understand well. The key, however, is being willing to change one’s views and approach based on new evidence. The application of the findings from these two studies, and many more that not infrequently conflict with each other is the biggest challenge for science communicators. It doesn’t help that we’re in the middle of a pandemic that has resulted in the spread of anti-science disinformation and conspiracy theories, many not innocently spreading but intentionally promoted. That a large amount of the disinformation that we see is not organic, but rather promoted by actors with agendas, just makes the problem even worse.
I don’t mean to finish on low note. The situation is not hopeless. In fact, it is research like this that is most likely to provide the tools to public health officials and science communicators to counter antiscience messages.